By Stephen DeAngelis
If you have paid any attention to the technology sector you are well aware of the ongoing battle between big tech companies to develop the most reliable large language model (LLM). You are also aware of concerns about the so-called hallucinations (i.e., false information) that can be generated by these models. Both the cost to use and the trustworthiness of LLMs have many CEOs wondering what’s best for their company. Author and keynote speaker, Dean DeBiase, explains, “For many companies, LLMs are still the best choice for specific projects. For others, though, they can be expensive for businesses to run, as measured in dollars, energy, and computing resources. … I suspect there are emerging alternatives that will work better in certain instances — and my discussions with dozens of CEOs support that [prediction].”[1] One of those alternatives is Small Language Models (SLMs). In many cases, SLMs are a better alternative than LLMs. In a recent LinkedIn post, I wrote, “There’s often a misconception that small language models are less effective than large language models — but the reality is, each serves different functions/needs based on the source data and information they use and the trustworthiness they bring.”
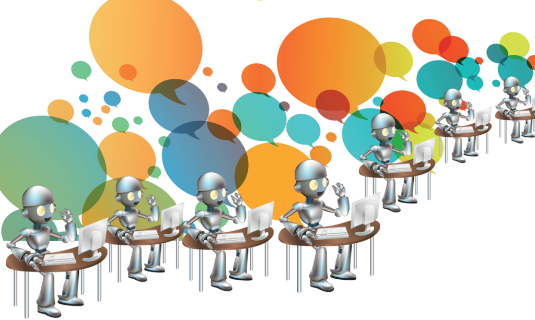
What Are Small Language Models?
The staff at SymphonyAI writes, “Small Language Models are specialized in specific tasks and built with curated, selective data sources. A small language model is a type of foundation model trained on a smaller dataset compared to Large Language Models. This focused training allows SLMs to learn the nuances and intricacies of specific domains, providing higher quality and more accurate results, increased computational efficiency, and faster training and development times.”[2] During a discussion between DeBiase and Steve McMillan, president and CEO of Teradata, McMillan noted, “As we look to the future, we think that small and medium language models, and controlled environments such as domain-specific LLMs, will provide much better solutions [for many companies].” Why? DeBiase explains, “A critical advantage of [SLMS is that] the data is kept within the firewall domain, so external SLMs are not being trained on potentially sensitive data. The beauty of SLMs is that they scale both computing and energy use to the project’s actual needs, which can help lower ongoing expenses and reduce environmental impacts.”
In my LinkedIn post, I stressed the importance of trustworthiness. I noted, “LLMs are not yet fully ‘Trusted,’ as they lack consistent alignment with known corporate or formal data/knowledge sources. SLMs are ‘trusted’ due to their use of data from recognized, corporate knowledge bases. … SLMs offer trusted, internally scalable and reliable solutions for companies of all sizes, designed to drive operational change.” DeBiase also stresses the importance of trustworthiness. He notes, “Because their knowledge is so broad, LLMs are also subject to hallucinations. These are responses that may sound correct at first, but turn out to be wrong (like your crazy uncle’s holiday party advice), often due to inapplicable or inaccurate information being used to train the models.”
Benefits of Small Language Models
DeBiase observes, “Since they don’t require the same gigantic clusters of AI-processing chips as LLMs, SLMs can run on-premises, in some cases even on a single device. Removing the need for cloud processing also gives businesses greater control over their data and compliance.” The SymphonyAI staff adds, “The primary advantage of small language models is their use of industry-specific training data. … By focusing on curated datasets relevant to each sector, these models can pinpoint nuances and intricacies crucial for accuracy. These models then power vertical AI applications designed to solve specific challenges for workers in each industry. Designed for users in each industry, this tailored approach provides immediate value to customers and allows for rapid innovation.” The team at Arthur notes the following benefits of SLMs when compared to LLMs.[3]
● SLMs are more lightweight & efficient: “This makes them more suitable for situations where computational resources are limited or where real-time inference is required.”
● SLMs are more accessible: “SLMs lower the barrier to entry for people who want to experiment with language models. Anyone who has access to a laptop or mobile device can train and deploy an SLM, whereas training and deploying an LLM would likely require expensive cloud services or specialized hardware.”
● SLMs are better for domain-specific tasks: “SLMs can be fine-tuned for specific domains or tasks, resulting in better performance and understanding within those particular areas.”
● SLMs are more secure: “Since SLMs have smaller codebases and fewer potential surfaces for security breaches, they are also less vulnerable to malicious attacks.”
● SLMs are better for the environment: “SLMs use less energy and memory than LLMs, which makes them more environmentally friendly and sustainable. They also have smaller footprints and faster inference times, which makes them more suitable for edge computing and real-time applications.”
The Arthur team also notes there are some drawbacks to SLMs. They observe that these drawbacks result from the fact that SLMs have fewer parameters, making them less flexible and less generally useful than LLMs.
Concluding Thoughts
DeBiase concludes, “SLMs can help businesses deliver better results. Although they have the same technical foundation as the well-known LLMs that are broadly in use today, they’re trained on fewer parameters, with weights and balances that are tailored to individual use cases. Focusing on fewer variables enables them to more decisively reach good answers; they hallucinate less and are also more efficient. When compared with LLMs, SLMs can be faster, cheaper and have a lower ecological impact.” I agree that small language models have the potential to level the playing field for many companies, especially in the logistics and retail sectors, because they are a more trusted, affordable and scalable solution. However, it’s important to remember that they aren’t simply plug and play solutions. Positioning any language model to perform AI-powered advanced analytics takes time and extensive training before they’re ready to tackle complex operational challenges. SLMs also need access to both real-time data and vast historical datasets to serve as industry-specific knowledge bases, as well as in-house AI expertise that can ensure they are trained and scaled effectively.
Footnotes
[1] Dean DeBiase, “Why Small Language Models Are The Next Big Thing In AI,” Forbes, 25 November 2024.
[2] Staff, “Small Language Model (SLM or SMLM),” SymphonyAI.
[3] Staff, “The Beginner’s Guide to Small Language Models,” Arthur Blog, 29 March 2024.